
Shared_codebook = True # whether to share the codebooks for all quantizers or not Sample_codebook_temp = 0.1, # temperature for stochastically sampling codes, 0 would be equivalent to non-stochastic You can use both of these features with two extra keyword arguments. The second is to stochastically sample the codes rather than always taking the closest match. The first is to share the codebook across all quantizers. # *_, (8, 1, 1024, 256) # all_codes - (quantizer, batch, seq, dim)įurthermore, this paper uses Residual-VQ to construct the RQ-VAE, for generating high resolution images with more compressed codes. Quantized, indices, commit_loss, all_codes = residual_vq(x, return_all_codes = True) # (1, 1024, 256), (1, 1024, 8), (1, 8) # (batch, seq, dim), (batch, seq, quantizer), (batch, quantizer) # if you need all the codes across the quantization layers, just pass return_all_codes = True Quantized, indices, commit_loss = residual_vq(x) Num_quantizers = 8, # specify number of quantizers
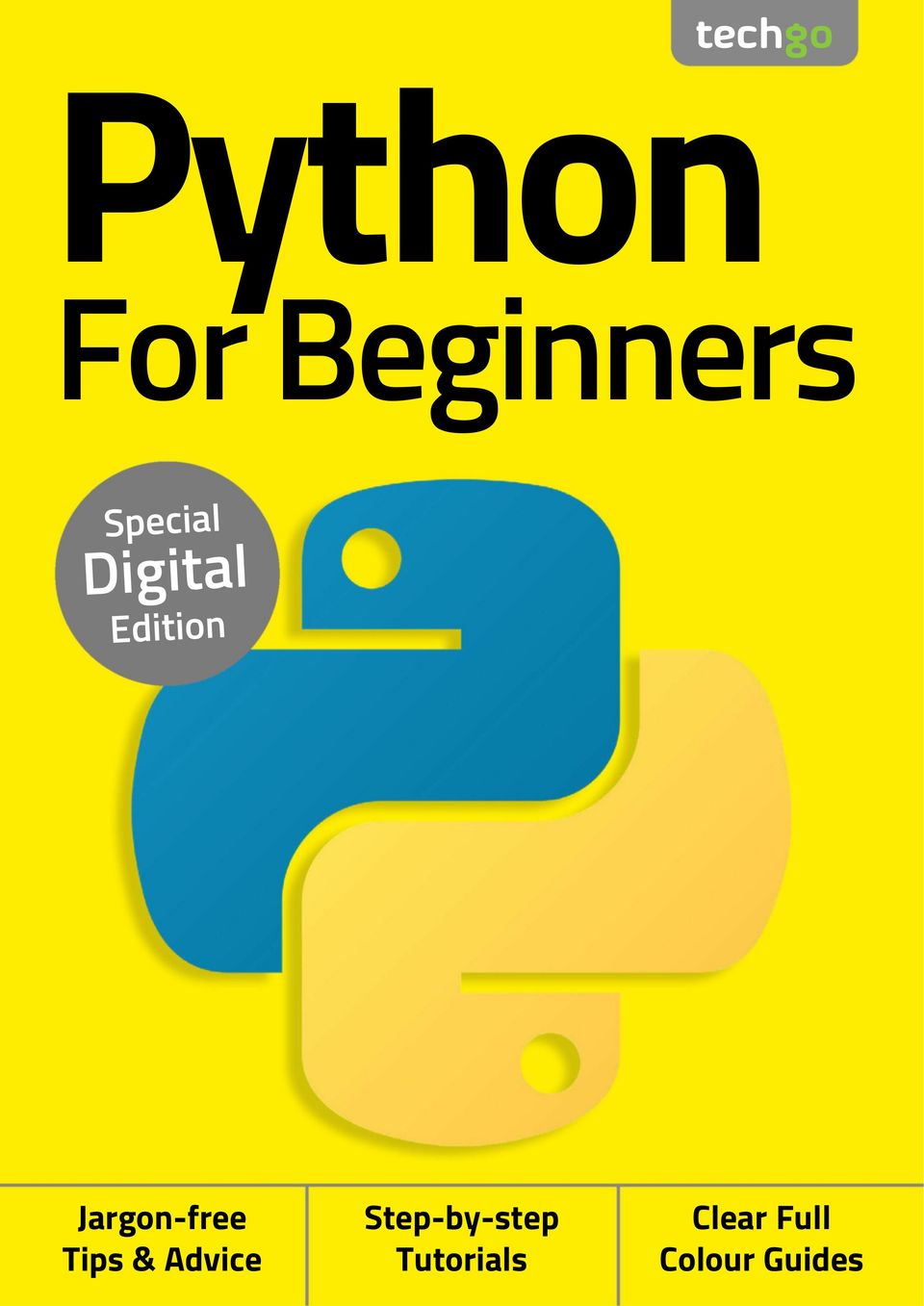
import torchįrom vector_quantize_pytorch import ResidualVQ You can use this with the ResidualVQ class and one extra initialization parameter. This paper proposes to use multiple vector quantizers to recursively quantize the residuals of the waveform.

#PYTHON CODEBOOK INSTALL#
Install $ pip install vector-quantize-pytorchįrom vector_quantize_pytorch import VectorQuantizeĭecay = 0.8, # the exponential moving average decay, lower means the dictionary will change fasterĬommitment_weight = 1.
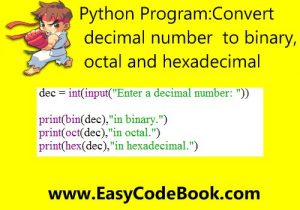
VQ has been successfully used by Deepmind and OpenAI for high quality generation of images (VQ-VAE-2) and music (Jukebox). It uses exponential moving averages to update the dictionary. A vector quantization library originally transcribed from Deepmind's tensorflow implementation, made conveniently into a package.
